Probabilistic Approaches for Transferring Human Skills to Humanoid Robots
Abstract:
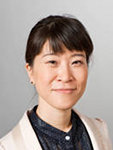
In this talk, recent research activities of Dynamic Human Robot Interaction lab at TUM will be presented, focusing on machine learning approaches for transferring human skills to robotic systems. Robot programming by demonstration provides an efficient way for a robot to learn new skills through human guidance, which can reduce time and cost to program the robot. Our mimesis model is inspired by the mirror neuron system and enables a humanoid robot to imitate whole-body motions from partially occluded data. The concept of whole body motion interpretation from partial observation is extended to 3D human motion capturing from a 2D image sequence and whole body motion association from tool knowledge. The inference mechanism can be applied not only to learn the robot's free body motion but also to learn physical interaction tasks such as grasping. In contrast to offline batch learning, unsupervised incremental learning techniques for segmentation and clustering are applied to enhance human-robot cooperation tasks over time with the special focus on prediction of human partner's behavior. The learned skills can be further improved by kinesthetic coaching, which leads to eliminating kinematic mapping errors and learning synchronized whole body motions. Finally the extension towards learning physical human robot interaction, where a robot companion is capable of handling intentional physical contacts with a human user, will be discussed. Direct physical interaction with a human during task execution is a widely undiscovered challenge. In our method, communication is designed in both symbolic and physical domains. The communication in the symbolic domain is realized through the concept of motion primitives and interaction primitives. In the physical domain, the trajectory of the motion primitive is reshaped in accordance with the human in real-time.